2025 Universal Cup Finals 赛事公告¶
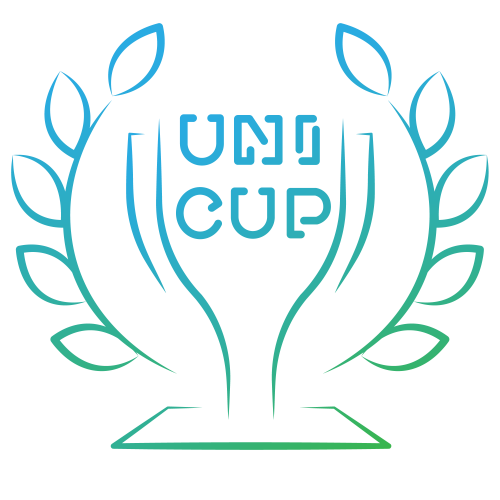
2025 Universal Cup Finals 将于 2.19 - 2.24 在中国东莞举办!
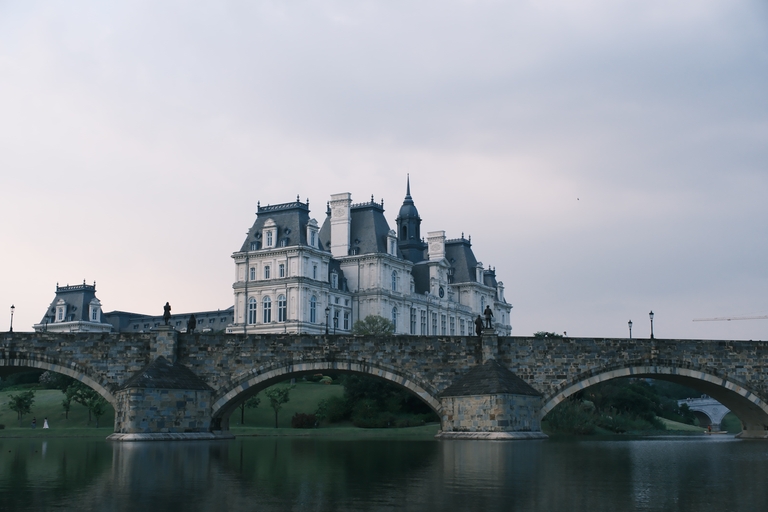
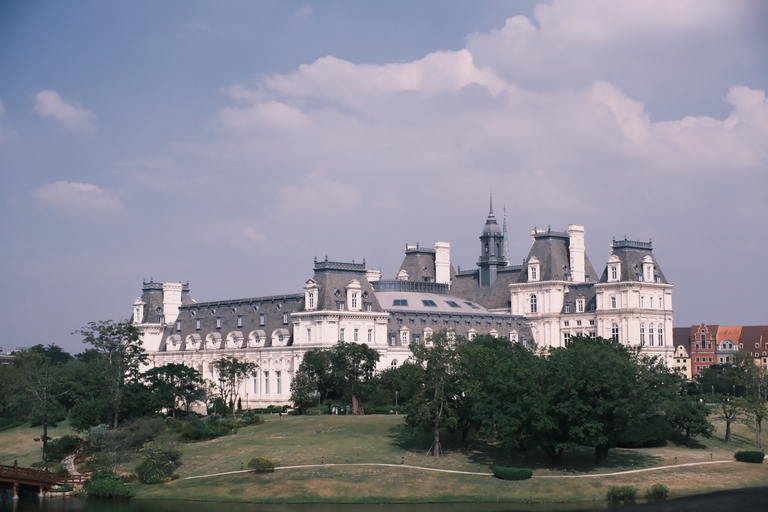
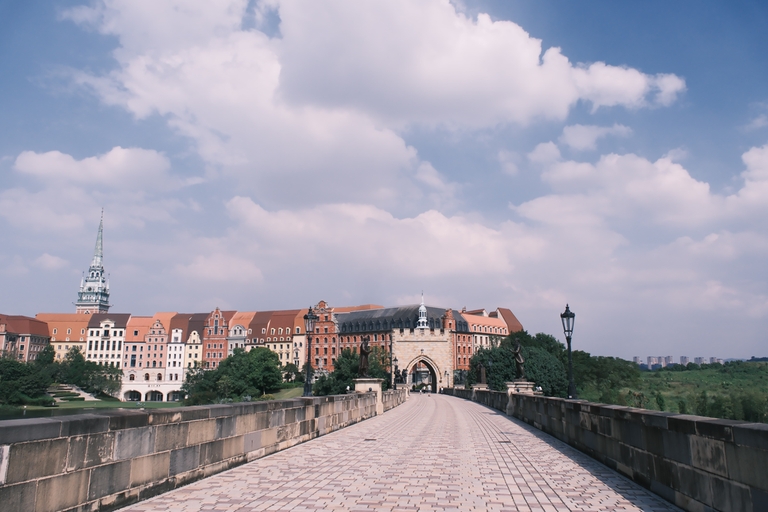
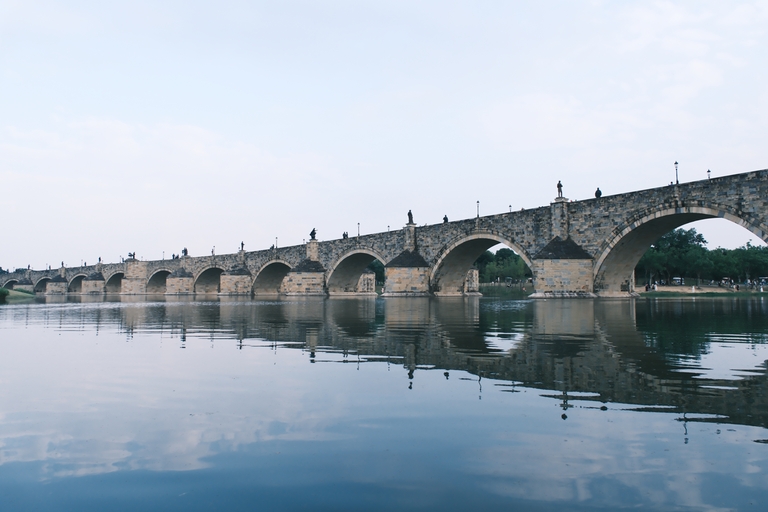
根据 2023 ~ 2024 年 Universal Cup 线上比赛以及第二届 Universal Cup Semifinals 的比赛结果, Universal Cup 科学委员会将根据 赛事规则 决定晋级决赛的队伍,邀请函将通过电子邮件发送。
Schedule¶
赛事日程如下:
Date | Topic | Arrangement |
---|---|---|
February 19 | Arrival | |
February 20 | Excursion | |
February 21 | Challenge Day | |
February 22 | Contest Day | |
February 23 | Conference Day | |
February 24 | Departure |
如果您有更多赛事相关的疑问,可以通过 [email protected] 联系我们。
Conference Information¶
Linear Systems Surprises¶
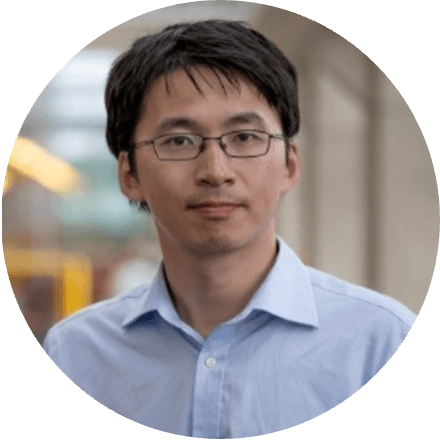
Presenter: Richard Peng (Carnegie Mellon University)
Abstract: Algorithms researchers strive to design better ways of solving problems that are central to many disciplines. Systems of linear equations arise throughout engineering and sciences in tasks ranging from physical simulation to data analytics. In many cases where linear systems don’t exactly model the problem, they provide the steps that lead to the solutions. Despite linear systems’ storied history spanning centuries, the current best algorithms for general linear systems, as well as many important subclasses, remain comparatively slow.
Over the last few decades, algorithms researchers developed entirely new approaches to solving linear systems. These progress led to accelerations in many applications, as well as entirely new theoretical frameworks for designing and analyzing algorithms. This talk will briefly overview some of the surprising ways of thinking about approximations, iterative convergences, and algebraic structures that originated from studying linear systems.
Practice on medical LLMs¶
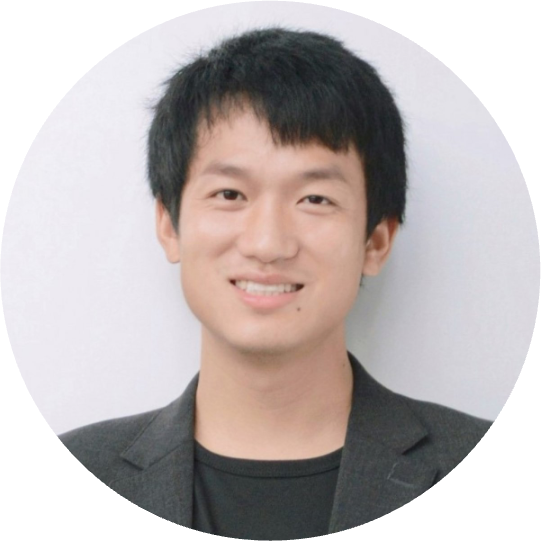
Presenter: Benyou Wang (The Chinese University of Hong Kong, Shenzhen)
Abstract: Recently, OpenAI's ChatGPT and various open-source community models, such as LLaMA 3, have significantly advanced the development of AI applications. In the medical field, both proprietary and open-source models hold great potential. However, when it comes to solving real-world medical problems, there is still a "last mile" to cover. In this Speech, we will introduce our team's development of the medical large language model, HuatuoGPT, and its multilingual and multimodal extensions, the Apollo series. We will also discuss the technical solutions for HuatuoGPT-o1, which aim to enhance the performance and interpretability of large language models, particularly in the context of longer diagnostic reasoning chains. Finally, we will look ahead to the future development of medical LLMs. Specifically, we will explore the potential of using AIGC technology to create a large number of patient agents to train both human and AI doctors. By doing so, we can accumulate real patient needs and doctor feedback, ultimately working towards the development of generalist medical artificial intelligence (GMAI).
Advancing Large Language Model Alignment through RLHF: Research Frontiers and Industrial Practices¶
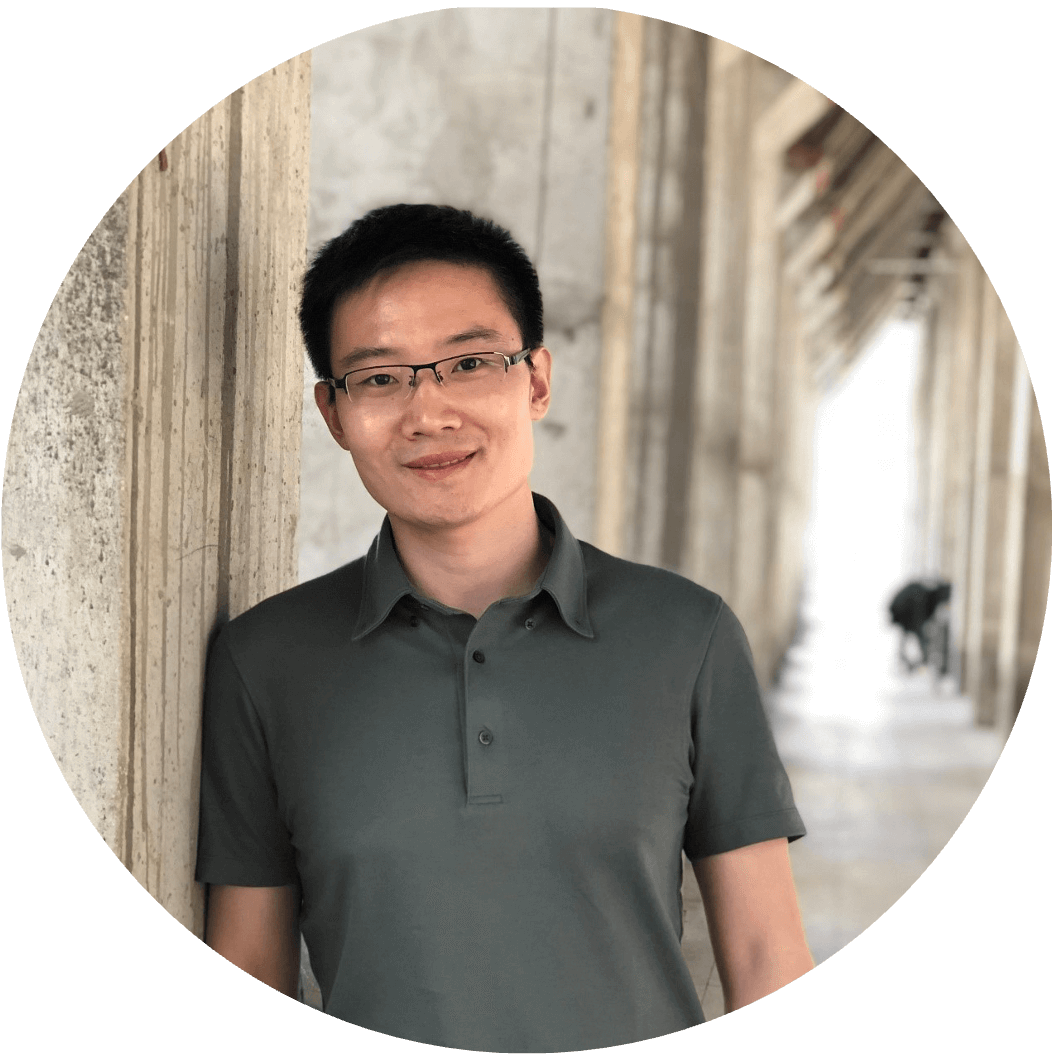
Presenter: Dong Li (Huawei Noah’s Ark lab)
Abstract: Large Language Models (LLMs) have emerged as a pivotal research focus across academia and industry. While substantial efforts have been dedicated to model pretraining, growing attention is being directed toward post-training optimization strategies, particularly reinforcement learning from human feedback (RLHF). This presentation systematically examines cutting-edge developments in RLHF methodologies, supplemented by implementation insights from Huawei's recent technical deployments. We further investigate next-generation alignment paradigms through the lens of slow-thinking architectures, exemplified by OpenAI's O1 and DeepSeek's R1 frameworks. We will share some interesting findings of slow-thinking model RL training.
An Introduction to Symbolic Program Synthesis¶
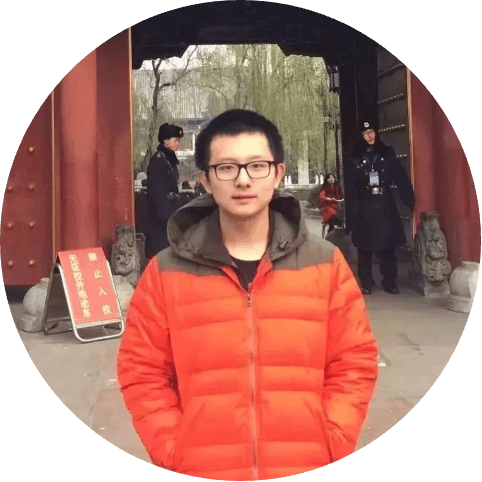
Presenter: Ruyi Ji (Peking University)
Abstract: Large Language Models (LLMs) have recently achieved remarkable success in program generation, particularly in competitive programming. For example, OpenAI reported that its o1 model achieved gold medal-level performance in last year's International Olympiad in Informatics (IOI), and its o3 model attained an estimated rating of ~2700 on Codeforces, ranking among top competitive programmers.
Despite these impressive milestones, current LLMs still suffer from several limitations. One major challenge is their inability to deduce general rules purely from examples, as their inference heavily depends on the presence of natural language. To address this limitation, symbolic approaches — representing a different paradigm of artificial intelligence — offer a complementary solution. Unlike LLMs, which rely on fitting vast amounts of data through large neural networks, symbolic systems represent knowledge via a small set of interpretable rules and perform reasoning by searching through combinations of these rules. While such systems are less effective at handling natural language, they excel at reasoning directly from structured examples.
In this presentation, I will provide an overview of symbolic program generation (i.e., program synthesis) and share recent progress in synthesizing efficient programs and complex algorithms.
Tight Bounds for Retrieval Data Structures¶
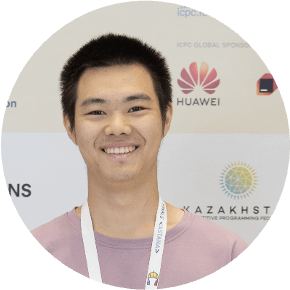
Presenter: Tingqiang Xu (Tsinghua University)
Abstract: Retrieval data structures are data structures that answer key-value queries without paying the space overhead of explicitly storing keys. The problem can be formulated in four settings (static, value-dynamic, incremental, or dynamic), each of which offers different levels of dynamism to the user. In this presentation, I will talk about optimal bounds for the final two settings (incremental and dynamic) in the case of a polynomial universe. This complete a line of work that has spanned more than two decades, and also come with a surprise: the incremental setting, which has long been viewed as essentially equivalent to the dynamic one, actually has a phase transition, in which, as the value size v approaches log n, the optimal space redundancy actually begins to shrink, going from roughly n log log n (which has long been thought to be optimal) all the way down to Θ(n) (which is the optimal bound even for the seemingly much-easier value-dynamic setting).